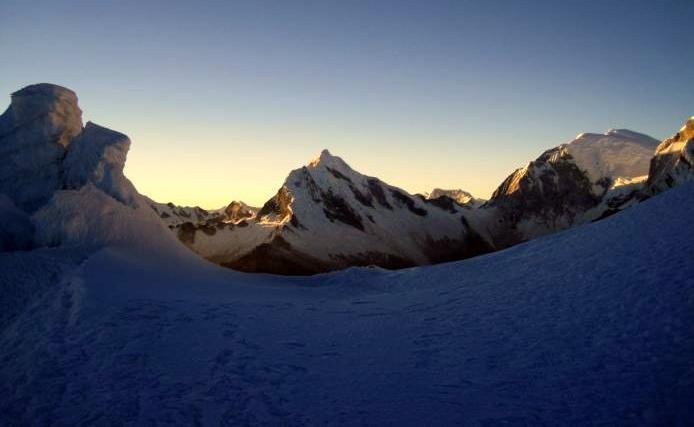
Atmospheric Downscaling for Glacierized mountain environments – DoG
Understanding and quantifying the response of mountain glaciers to changing climate and weather conditions is a pressing task for climate scientists and glaciologists all over the world. To date, models that relate glacier mass and dynamics to atmospheric variations are well established. It has remained as a major challenge, however, to accurately describe the past, present and future atmospheric states that affect the glaciers. DoG systematically investigates the following research hypothesis: “The uncertainty in global and regional glacier simulations can be reduced significantly by reducing the uncertainty in the atmospheric drivers of the glacier simulations.”
To test this hypothesis, DoG focuses at the development, application and rigorous validation of a state-of-the-art downscaling framework for glacierized mountain environments situated in a diversity of climatic and geo-environmental settings. This downscaling framework contains elements of the most modern statistical, as well as so-called hybrid downscaling techniques applied to reanalysis data predictors, for all atmospheric target variables required in process-based glacier mass balance models. The performance of the available global reanalysis data sets will be assessed for the purpose of glacier mass modelling, on a regionally differentiated view. Using in situ observations from stations near to- and on the glaciers, the transfer of the downscaling framework to glacierized mountain sites in various topographic and climate settings will be validated. This validation procedure pays particular attention to appropriately consider the pitfalls of short or patchy observation records that are often the only data source in remote mountains.
The DoG database: number of stations participating in the DoG project, within each RGI region. The circles are proportional to the areas of the glaciers within each RGI region (shown in orange)
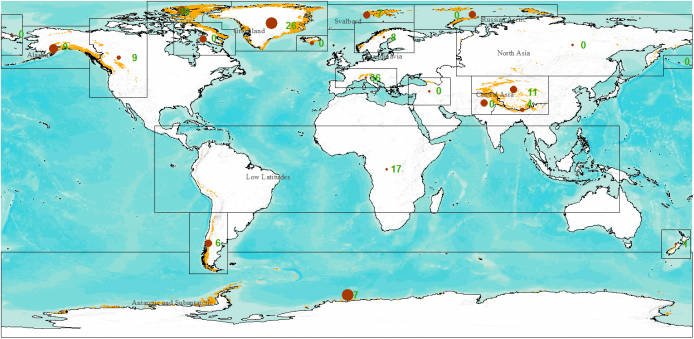
NEWS: Call for AWS data – DoG
We call for data from automatic weather stations situated in the vicinity of mountain glaciers all over the world, to be included in the DoG project.
Data series considered by the DoG project include
- data from stations situated on, or near (at less than half a kilometer distance from) mountain glaciers (stations situated farther away from the glaciers will probably be considered for very data-sparse regions),
- all variables required for process-based glacier mass modeling, in particular air temperature, relative humidity, wind speed, precipitation, and global radiation,
- at daily and preferably higher time resolutions,
- of minimum 2 years record length, and
- data which are not in the operational archives of the major weather forecasting centers (e.g., ECMWF).
Removal of unreliable data should ideally appear as gaps in the data series, especially if this concerns larger parts of the data.
All data contributors will be kept updated about publication activities with their data involved. The data will not be shared outside the project.
Glaciologists and climate scientists from around the world with interest in including their study site in the DoG experiment are invited to get in contact with Marlis.Hofer@uibk.ac.at, Fabien.Maussion@uibk.ac.at or Johannes.Horak@uibk.ac.at
Project Leader:
Marlis HOFER
Fabien MAUSSION
Members:
Horak Johannes
Co-PI:
Georg KASER
Ben MARZEION
Funding Agencies:
Austrian Science Fund (FWF) P28006
Project Duration:
01/06/2015 to 31/05/2020